In the age of data-driven decision-making and artificial intelligence (AI), the quality of data used is paramount. Just as junk food can harm our physical health, can have detrimental effects on the performance and outcomes of AI systems. This article explores the concept of bad data, its implications on AI, and offers solutions to ensure the data consumed by AI is accurate, reliable, and of high quality.
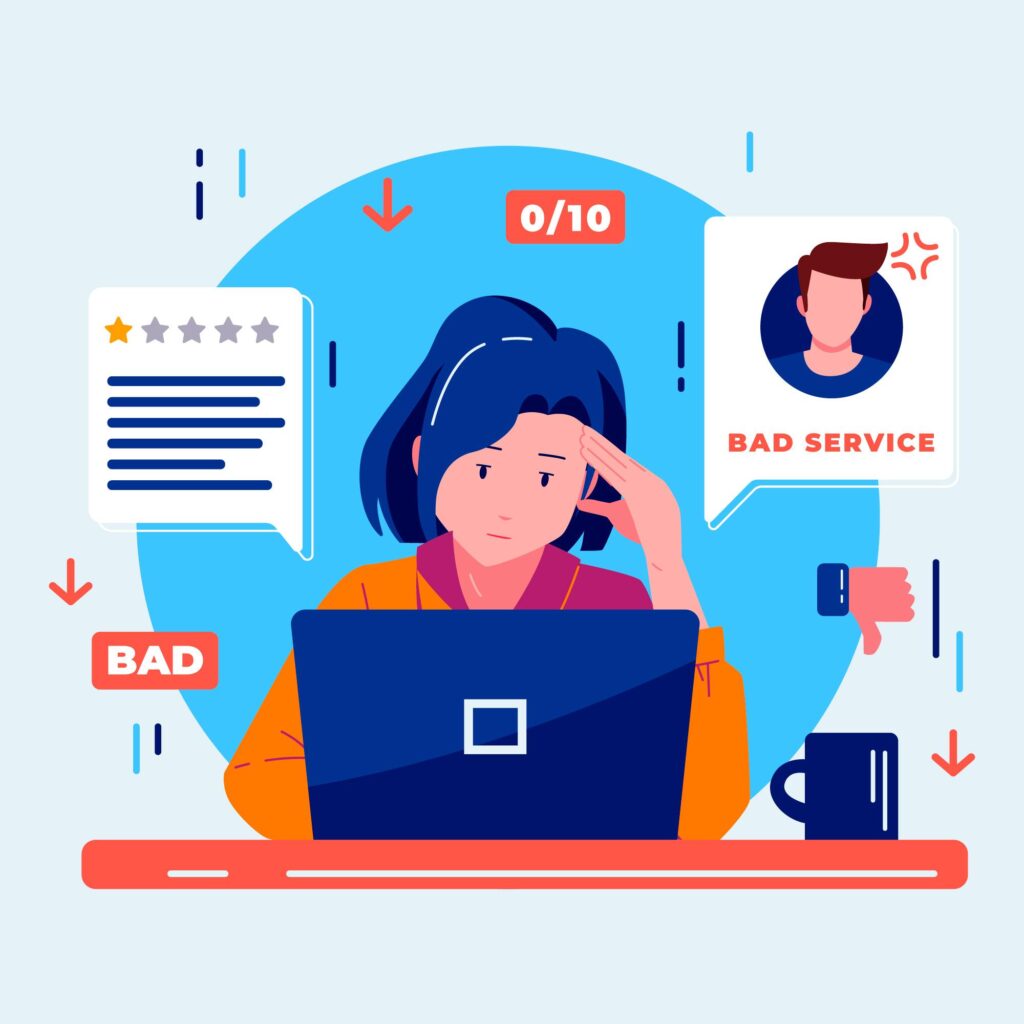
Defining Bad Data
Bad data refers to inaccurate, incomplete, inconsistent, or misleading information. It can stem from various sources, such as human error, data entry mistakes, outdated records, or system glitches. When it infiltrates AI systems, it hampers their ability to make reliable predictions, impairs decision-making processes, and undermines the integrity of the AI-driven operations.
Implications on AI
a. Decreased Accuracy: Bad data compromises the accuracy of AI models, leading to flawed predictions and unreliable outcomes. Garbage in, garbage out (GIGO) becomes a reality, as AI algorithms can only work with the data they are fed.
b. Biased Results: It can perpetuate biases present in the data set, leading to biased AI models. If the input data is skewed or incomplete, AI systems may reproduce and amplify existing biases, resulting in unfair or discriminatory outcomes.
c. Inefficient Resource Allocation: AI models trained on bad data can misguide resource allocation decisions, wasting valuable time, money, and efforts. Flawed insights derived from it can lead to misguided marketing campaigns, ineffective product recommendations, or poor customer service.
d. Damaged Customer Trust: When AI-powered systems produce inaccurate or unreliable results, it erodes customer trust. Unreliable predictions, incorrect recommendations, or flawed personalized experiences can damage customer relationships and tarnish a brand’s reputation.
Identifying and Mitigating
a. Data Validation and Cleansing: Implementing robust data validation processes ensures that only accurate and reliable data enters the AI system. This includes performing integrity checks, removing duplicates, correcting errors, and validating data against predefined criteria.
b. Data Governance: Establishing data governance frameworks, including data quality standards, data lineage, and documentation, helps ensure data integrity and accountability throughout the organization. Clear guidelines and protocols for data collection, storage, and maintenance minimize the risk of bad data infiltrating AI systems.
c. Feature Engineering and Selection: Proper feature engineering, where relevant and meaningful data attributes are selected, is crucial to avoid noise and irrelevant information. Careful feature selection enhances the accuracy and performance of AI models.
d. Continuous Monitoring and Feedback Loop: Implementing a feedback loop that monitors AI system performance and collects user feedback enables ongoing data refinement and model improvement. By incorporating human oversight, organizations can detect and rectify issues caused by bad data in real-time.
e. Ethical Considerations: Adhering to ethical guidelines and regulations in data collection and usage is essential. Organizations must ensure fairness, transparency, and accountability in AI systems to mitigate biases and prevent the negative impact of bad data on marginalized groups.
Importance of Data Quality Culture
Cultivating a data quality culture within organizations is crucial. By promoting data literacy, encouraging responsible data practices, and fostering a mindset that values data accuracy and integrity, organizations can prevent bad data from infiltrating AI systems. Training and educating employees about data quality best practices and the importance of data governance are key steps towards building a data-driven culture.
Collaboration between Data Scientists and Domain Experts
Collaboration between data scientists and domain experts is vital to ensure the accuracy and relevance of AI models. Domain experts possess deep knowledge and contextual understanding that can help identify and address potential issues related to bad data. By involving domain experts in the data collection, preprocessing, and validation processes, organizations can mitigate the risks of it and improve the overall quality of AI-driven insights.
Leveraging AI for Data Quality
Paradoxically, AI can also be leveraged to improve data quality. AI-powered algorithms can assist in data cleansing, anomaly detection, and data validation processes. By utilizing AI tools specifically designed for data quality enhancement, organizations can automate the identification and correction of bad data, leading to more reliable and accurate datasets for AI training and decision-making.
Continuous Improvement and Learning
Data quality is an ongoing process that requires continuous improvement and learning. Organizations should regularly assess their data quality practices, monitor the performance of AI models, and adapt to evolving technologies and best practices. By staying updated and proactive, businesses can ensure that their AI systems are built on a foundation of high-quality data.
Bad data is like junk food for your AI—it hampers its performance, undermines decision-making, and erodes trust. Recognizing the implications of bad data on AI systems is crucial for organizations seeking reliable and accurate insights. By implementing strategies such as data validation and cleansing, data governance, feature engineering, continuous monitoring, and fostering a data quality culture, organizations can mitigate the risks associated with it. Collaboration between data scientists and domain experts, leveraging AI for data quality improvement, and maintaining a commitment to continuous learning are key to ensuring the accuracy and reliability of AI-driven outcomes. By investing in high-quality data, organizations can unlock the full potential of AI, make informed decisions, and drive sustainable growth in the data-driven era.
Related Articles: